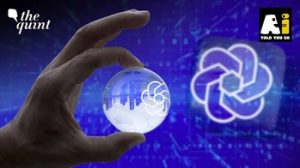
As generative artificial intelligence (AI) innovation continues at a breakneck pace, concerns around security and risk have become increasingly prominent. Some lawmakers have requested new rules and regulations for AI tools, while some tech and business leaders have suggested a pause on training of AI systems to assess their safety.
Here, Avivah Litan, VP Analyst at Gartner, discusses what data and analytics leaders responsible for AI development need to know about AI trust, risk and security management.
Given concerns around AI security and risk, we may wonder whether organisations are continuing to explore the use of generative AI, or whether a pause is warranted. The situation is fairly clear according to Avivah Litan, who suggests that in reality, generative AI development is not stopping. “Organisations need to act now to formulate an enterprise-wide strategy for AI trust, risk and security management (AI TRISM). There is a pressing need for a new class of AI TRISM tools to manage data and process flows between users and companies who host generative AI foundation models.”
“There are currently no off-the-shelf tools on the market that give users systematic privacy assurances or effective content filtering of their engagements with these models, for example, filtering out factual errors, hallucinations, copyrighted materials or confidential information. AI developers must urgently work with policymakers, including new regulatory authorities that may emerge, to establish policies and practices for generative AI oversight and risk management.”
Avivah Litan says generative AI raises a number of new risks that organisations should take very seriously. She explains that so called “hallucinations” and fabrications, including factual errors, are some of the most pervasive problems already emerging with generative AI chatbot solutions. Training data can lead to biased, off-base or wrong responses, but these can be difficult to spot, particularly as solutions are increasingly believable and relied upon.
“Deepfakes, when generative AI is used for content creation with malicious intent, are a significant generative AI risk. These fake images, videos and voice recordings have been used to attack celebrities and politicians, to create and spread misleading information, and even to create fake accounts or take over and break into existing legitimate accounts.”
Litan quotes a recent example. “An AI-generated image of Pope Francis wearing a fashionable white puffer jacket went viral on social media. While this example was seemingly innocuous, it provided a glimpse into a future where deepfakes create significant reputational, counterfeit, fraud and political risks for individuals, organisations and governments.”
Data privacy is a further issue highlighted by Avivah Litan. “Employees can easily expose sensitive and proprietary enterprise data when interacting with generative AI chatbot solutions. These applications may indefinitely store information captured through user inputs, and even use information to train other models — further compromising confidentiality. Such information could also fall into the wrong hands in the event of a security breach.
Also copyright issues. Generative AI chatbots are trained on a large amount of internet data that may include copyrighted material. As a result, some outputs may violate copyright or intellectual property (IP) protections. Without source references or transparency into how outputs are generated, the only way to mitigate this risk is for users to scrutinise outputs to ensure they don’t infringe on copyright or IP rights.”
“In addition to more advanced social engineering and phishing threats, attackers could use these tools for easier malicious code generation. Vendors who offer generative AI foundation models assure customers they train their models to reject malicious cyber security requests; however, they don’t provide users with the tools to effectively audit all the security controls in place. The vendors also put a lot of emphasis on “red teaming” approaches. These claims require that users put their full trust in the vendors’ abilities to execute on security objectives.”
However there are actions that enterprise leaders can take now to manage these generative AI risks. It is important to note that there are two general approaches to leveraging Chat GPT and similar applications. “Out-of-the-box model usage leverages these services as-is, with no direct customisation. A prompt engineering approach uses tools to create, tune and evaluate prompt inputs and outputs,” continues Litan. “For out-of-the-box usage, organisations must implement manual reviews of all model output to detect incorrect, misinformed or biased results. Establish a governance and compliance framework for enterprise use of these solutions, including clear policies that prohibit employees from asking questions that expose sensitive organisational or personal data.”
She also advised that organisations should monitor unsanctioned uses of Chat GPT and similar solutions with existing security controls and dashboards to catch policy violations. For example, firewalls can block enterprise user access, security information and event management systems can monitor event logs for violations, and secure web gateways can monitor disallowed API calls.
“For prompt engineering usage, all of these risk mitigation measures apply. Additionally, steps should be taken to protect internal and other sensitive data used to engineer prompts on third-party infrastructure. Create and store engineered prompts as immutable assets. These assets can represent vetted engineered prompts that can be safely used. They can also represent a corpus of fine-tuned and highly developed prompts that can be more easily reused, shared or sold,” concludes Avivah Litan.
Gartner analysts will be discussing AI TRISM at the Gartner Security & Risk Management Summits taking place in the US, Japan and the UK, in June, July and September, respectively.