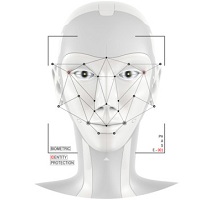
Facial Recognition has received a significant amount of press over the last several years, and with significant advancements in available processing power and artificial intelligence, there is no sign that it will abate. Not all facial recognition is created equal, however, so let’s dive into it a little more deeply. Simply put, facial recognition is the use of distinguishable facial features for the purpose of identifying and, possibly, authenticating a person. Whatever means is used to profile that face leads to the creation of a binary data file that becomes the standard for comparison for later operations. If you think about it, facial recognition was initially a manual process where the standard for comparison was embedded in someone’s memory or a compilation of photos, but relied on human judgment to decide. Different types of facial biometrics are provisioned for static (stationary) vs. dynamic (in motion) applications.
Static Applications
Static applications normally start out with a still photo of varying resolution. The photo is most often a digital file, where some degree of jpeg compression – depending on required resolution – is employed. Automated comparison on a straight jpeg file is extremely difficult and quite impractical, because a decision rule must be employed based on number of matching pixels, following some type of processing and formatting so that a reasonable comparison can be made.
The more efficient means of analysis is to examine the picture using various reference points to get to some degree of normality, extract the key parameters (such as distance between the eyes) and digitize those significant elements into files of limited size. Decision rules still must be applied with thresholds for acceptance and rejection. Even so, there is the opportunity for systems to be spoofed and defeated, such as a third party holding a picture of the authenticated person in front of the camera.
One system produced by Stone Lock Global, effectively addresses these practical concerns. Its Stone Lock Pro system employs near-infrared (NIR) light to sample more than 2,000 points on a face – measuring the reflected energy from each point. Because NIR actually penetrates a short distance below the skin surface, the points have characteristically different reflective properties and generate different values based on reflectance.
The resulting profile has been proven to be unique and non-spoofable, even with identical twins. The system will work in darkness, but is not designed for outdoor use. Scanning more intensely in certain areas of the face leads to the establishment of ”reference points” that make the process reproducible for subsequent scans.
Measurements are encapsulated in a 38 kB file, which is then encrypted and stored in a Biometric Signature Data (BSD) file, which contains no personally identifiable information. Decryption and verification are performed with Stone Lock Pro’s proprietary algorithms, and are only usable to verify an enrolled user. Its False Acceptance Rate (FAR) is <0.0004%, although there have been no known spoofs of the system to date.
Dynamic Applications
Taking on the in-motion challenge is FST Biometrics, based in Israel. Again, FST starts out with a fixed, high-resolution picture. Using various facial features, a set of “vectors” is derived and contained in a 2 KB file. The primary FST use case is grabbing multiple images from a video feed and creating vectors from each face to compare against the database of possible vectors (N:N). This is computationally intensive, and it is very difficult; yet, for this in-motion application, FAR of <.03% and False Rejection Rate (FRR) of<.2% are achieved. The result of a false rejection may just require another pass before the camera.
One of the key elements that contributes to the system’s reliability is an advanced artificial intelligence-based anti-spoofing algorithm, officially approved in the U.K., that prevents using any type of picture – color, B&W or IR – from spoofing the system. Furthermore, FST does not rely solely on the facial vector comparison to make their In Motion Identification system operate successfully.
The use of artificial intelligence and deep learning is leading to smarter systems that learn as they go along. FST learns “body behaviors” extracted from the visual data it is constantly accumulating. Physical attributes and personal tendencies are accumulated into their own vectors that, along with the facial vector, form a user record. These vectors work together in a flexible way to make a decision on a match.
The Future of Facial
In the future, facial recognition will evolve from identification and authentication to monitoring certain types of behaviors. For example, General Motors has announced a system that will monitor a driver’s face to determine if he/she is actually paying attention to the road while the vehicle is in cruise control.
Imagine the possibilities as behavioral analytics evolves, where a system could differentiate what it has learned to be normal facial expressions from those that are abnormal. Then factor in learned body behaviors. This “learning” is made possible by recent advances in processing power and the tools that allow those processors to learn over time as they encounter more data. Not only does this create exciting new possibilities for surveillance and public safety applications, business ramifications will also become apparent.