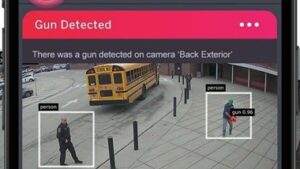
Omnilert, a pioneer in active shooter solutions, has announced the release of its third-generation AI visual gun detection system, featuring significant advancements designed to further advance the industry’s most effective gun detection system.
As the only solution to deliver a unique combination of detection, verification, activation, and notification, Omnilert Gun Detect protects hundreds of schools, universities, hospitals, retail and commercial buildings, and other organisation facilities and campuses around the country.
The enhancements made in this new release increase the company’s technology leadership with hardware efficiency, enterprise management, and gun detection accuracy. Omnilert Gun Detect is an AI-powered visual gun detection software that delivers reliable, 24/7 monitoring of gun threats using existing security cameras.
The system can identify a weapon in a fraction of a second, Omnilert Monitoring can verify in as little as three seconds and then activate a response in only two more seconds that can include dispatching police, locking doors, sounding alarms, and automating other responses to notify those in harm’s way and save lives.
One of the unique capabilities of the system is that it sends both still images and a short video clip when a threat is detected to provide more context to security personnel and first responders.
During an event, it can also deliver ongoing situational awareness about the location and details of the shooter so important decisions can be made based on the timeliest data.
With the third generation of this system, Omnilert has added several key enhancements that provide customers with effective, efficient, and accurate AI visual gun detection technology that can be easily added as a vital layer of their overall security efforts.
Omnilert Gun Detect’s AI architecture has been optimised to boost performance 10X for any given GPU over its original architecture, enabling more camera streams to be processed per server. Benefits of these enhancements include:
● Lower hardware costs for customers.
● High-performance processing on highly available, commodity GPU and server platforms.
● (55) 1080P camera streams running at 15fps are achievable on a standard NVIDIA RTX 4060.
● Architecture efficiency for ensuring high frame rate video processing, providing 3-5X more opportunities to detect a gun every second vs. other solutions on the market.
● System operations occur at the provided frame rate in real-time, instantly processing Variable Frame Rate streams from 1FPS to 30FPS without any delay in detection capability.
● PTZ (pan, tilt, zoom) camera compatibility due to real-time frame rate analysis.
New clustering enhancements to Omnilert Gun Detect ensure enterprise deployment to tens of thousands of cameras across thousands of sites and servers manageable from a central console. Benefits of this include:
● Simplified management of large deployments across school districts, healthcare systems, and throughout entire retail, commercial, government, and corporate enterprises.
● Easily allows for centralised, dense rack server deployments in data centers to decentralised site-level server or appliance installations or a combination to accommodate a variety of networking topologies.
The Omnilert Gun Detect neural network model is in its 38th revision, with continual learning and refinements from curated organic video through real-world customer deployments over its five years of development and fine-tuning.
This accuracy is unmatched in the industry compared to solutions created from image datasets that rely on synthetic/green screens or emulated data for training which produces artefacts not found in real life. This approach reduces the system’s ability to be tuned or discern the difference between objects, resulting in higher false positives, more noise, and significant operational burden irrespective of who is performing the verification function.
Data-centric AI model
In contrast, Omnilert’s proprietary data-centric AI model does not simply compare objects to a database of known gun images. It is based on raw video footage captured directly from security cameras in various real-life settings around the world and reflects the genuine complexities and nuances of everyday environments such as changing lighting conditions to specific human behaviours. This ensures higher detection accuracy and efficiency in processing which translates into lower hardware requirements.