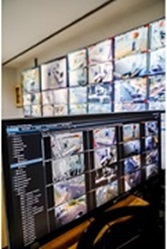
These days there is a general awareness amongst security professionals that the software and video cameras needed for analytics can be expensive. These costs are often highlighted within articles that appear in related search results. But in this article, Milestone experts talk about the lesser-known (hidden) costs of analytics.
Security cameras have come a long way. Some of them have basic built-in analytics. One example is line crossing, where an alert goes out when a moving object crosses a user-defined line (e.g., someone jumping over a fence). But even with basic analytics, there’s no such thing as “plug and play”.
Milestone suggests a couple of points that vendors can fail to mention :
● Even if your cameras can handle the analytics, someone will need to log into each camera interface at a time to get it set up. Unless you want to pay for a separate tool that lets you deploy everything in bulk.
● Once the analytics have been running for a little while, you’re going to need to go back and fine-tune each camera’s settings, placement, angles and detection range.
● If you’re a seasoned veteran in the world of security, these parts of the job will likely be familiar. But they can still be easy to overlook when drafting a realistic budget.
● Advanced analytics need (expensive) servers
If we additionally consider examples of more heavy-duty analytics for example, occupancy monitoring which needs to combine data from several cameras, or AI license plate recognition and facial recognition which rely on databases. And heat mapping is dependent on historical data. All of these need to run on a server rather than on a camera. At this point we question how expensive this could all get, but that depends on varying factors.
According to Milestone, the bulk of the server room costs will come from storage requirements, so that’s an important budget line to highlight.
While video management software (VMS) solutions such as the Milestone Xprotect can perform analytics such as forensic search on the server side of things, organisations use separate tools for analytics for, say, facial recognition. These tools have specific processor and RAM requirements, so it’s worth shopping around.
Another way to cut down costs is to consider what proportion of your site actually needs the heavy-duty stuff. For example when considering a project in an airport, you might want facial recognition software coverage across all spaces in all terminals. This would let you search for a known face among the crowds and get a recorded video of the person of interest presented as a timeline. But it would also cost quite a lot.
Some airports decide to keep costs down by only having facial recognition in the passport check/immigration area. They might run analytics on supercomputers for 100 cameras dedicated to this priority area. Meanwhile, their remaining 1,900 security cameras around the airport might rely on less resource-intensive analytics on lower-budget servers.
Training the machines for machine learning
While the promise of machine learning is enticing, there is a conspicuous absence of people asking who will teach the algorithms. Let’s say a manufacturing company wants to implement personal protective equipment (PPE) detection. In other words, they want their cameras and deep-learning algorithms to work together to automatically identify and notify them when someone isn’t wearing the mandatory helmet, safety boots, gloves, etc.
But software isn’t always good at telling the difference between a hat and hair, or differentiating between normal clothing and protective gear if the colours and shapes are similar. The initial functionality depends a lot on how much time and care a real live person (or people) spent 1) installing cameras for optimal coverage and visibility, and 2) training the algorithm on examples of proper PPE versus PPE violations. Regardless, false alarms will happen. And mistakes will be found and reported by humans; a machine won’t know about its mistake until feedback is manually entered.
In this instance then there will be costs involved for calibration services. In other words, someone who can analyse the data, identify the reason(s) behind false detections and mitigate the underlying issues. This usually consists of a minimum of two visits; one for analysis and initial implementation of improvements, and one for comparing the results and reprocessing. Whether these “teaching” services are hired in-house or outsourced, a cost is a cost.
The VMS can impact the cost of analytics
Milestone VMS, Xprotect, is engineered to make the most of metadata. The efficient Search function lets operators quickly find evidence of specific events, objects and/or people. This type of analysis takes place on the server side, as it relies on reviewing video from multiple cameras and so can help cut costs.
When it comes to working with third-party analytics like facial recognition, the hardware requirements of Xprotect are, according to Milestone, almost always lower than alternative options and usually by a significant factor.
In addition, it sometimes happens that VMS vendors charge extra for integrating with third-party analytics but Milestone does not charge extra for integrating with Xprotect.
The Xprotect open platform design allows the user to combine analytics from different camera brands at no additional cost. Open platforms are also useful for server-side analytics. This means the client is not locked into any particular analytics tool, as Xprotect works with pretty much everything.
This offers the flexibility to start off with camera-side analytics and potentially wait until the next budget period to invest in third-party server-side analytics. This also presents more time to decide how many cameras will need the more expensive analytics.