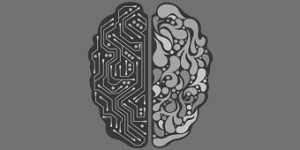
A recent incident involving Facebook revealed insights into its research experiment with chatbots. As it happened, two chatbots developed their own language and started to communicate with each other, which had to be shut down immediately, possibly because they had deviated from the script.
While this sounds amusing, it illustrates how the years of academic research has paid off, and we now have artificial intelligence (AI) systems that can learn. AI may be on the cusp of broad adoption, but this creates both opportunity and risk. A brief history of AI helps to clarify how it has become a phenomenon today, with common applications that include computer vision, natural language processing, speech recognition and data mining. In the 1950s, scientists laid the theoretical foundation for AI, but they did not have the computing power to build practical applications. In the late 1980s, funding for this research dried up.
However, in 1997 the Deep Blue supercomputer from IBM beat the reigning chess champion and having reignited interest in AI, the turn of the millennium saw the emergence of robotics amid advances in sensor technology. Very recently, the emergence of neural networks has proven to be better than humans in tasks such as visual object recognition.
Clearly, the history of AI is one of success and failure. So, the important question now is: what is powering the progress in AI? The answer has three main components.
The first is the availability of computing power, in the Cloud and GPUs. Secondly are the advances in machine learning, particularly neural networks. The third is sensor fusion. Sensors are cheaper, faster and more prolific than ever.
Looking at research from prominent analysts, plans for mass adoption are very promising. Gartner estimates that AI is about two-five years away from mainstream adoption, for instance, and 75% of executives polled by the Economist Intelligence Unit think AI is so important they plan to implement in their business within the next three years. Understanding the potential of AI and how it will be used in the security industry begins with the formative efforts of early pioneers. The low-hanging fruit is data mining using machine learning; for example, with loss prevention in department stores, grocers, wholesalers, etc.
To predict loss prevention, retailers can identify variables that are highly correlated to shrink. Those variables fall into four categories: financial, merchandising, human resource and point-of-sale (PoS). The machine learning technique is regression modeling by which an equation is created to reflect the correlation between shrink and variables, such as refunds, inventory balance and voided transactions at PoS. Data-driven retailers are die-hard fans of this technique and report savings in the millions of dollars and rapid return on investment.
Video analytics is another area where AI techniques, such as deep learning algorithms, are broadly applied. In the security industry a number of vendors provide “black box” video analytics that can be used for nonscan detection at PoS and object recognition, but the big tech vendors are taking this further by offering cognitive services in the Cloud.
Microsoft offers a service on the web that takes an image and then returns a JSON file showing its analysis. For example, given a group photo, the system can detect faces, score emotions and return an analysis of group emotion. Cognitive services like this are going to be more common in the future. They could be used, for example, at a bank’s branch office to return a benchmark of customer satisfaction based on the collective emotion reflected in the faces of customers.
Other virtual assistants exist, too, and can perform functions such as following up on leads through email. Google and Amazon are coming up with powerful products such as Lex and Dialog Flow, which are worth keeping an eye on. A lot is happening in the robotics space too, including security guard duty in this industry. Elsewhere, companies have been experimenting with robots as greeters in their mobile stores. According to their designers, the robots are capable of human interaction and empathy.
Post beta testing, the retailers’ report increased foot traffic in stores. Robots are also being used in logistics and delivery. Amazon continues to experiment with delivery via drones, as has been well documented. Even Domino’s has begun using a self-driving DRU (Domino’s Robotic Unit) in some parts of the world to deliver pizza!